Artificial intelligence (AI) refers to digital computers and robots that exhibit intellectual processes typical of human beings. This includes the ability to learn and generalize from past experience.
However, implementing and maintaining AI workflows has operational risks like model drift, bias and breakdowns in governance. And security vulnerabilities that threat actors can use to attack AI systems pose additional threats. Find out more information at NSFW AI Roleplay.
The origins of AI
Science fiction and early research into calculating machines familiarized the world with artificial intelligence. Many researchers had a starry-eyed belief in the technology’s imminent arrival. They envisioned a machine capable of understanding language and other entities, making decisions, and solving problems—all without any assistance from humans.
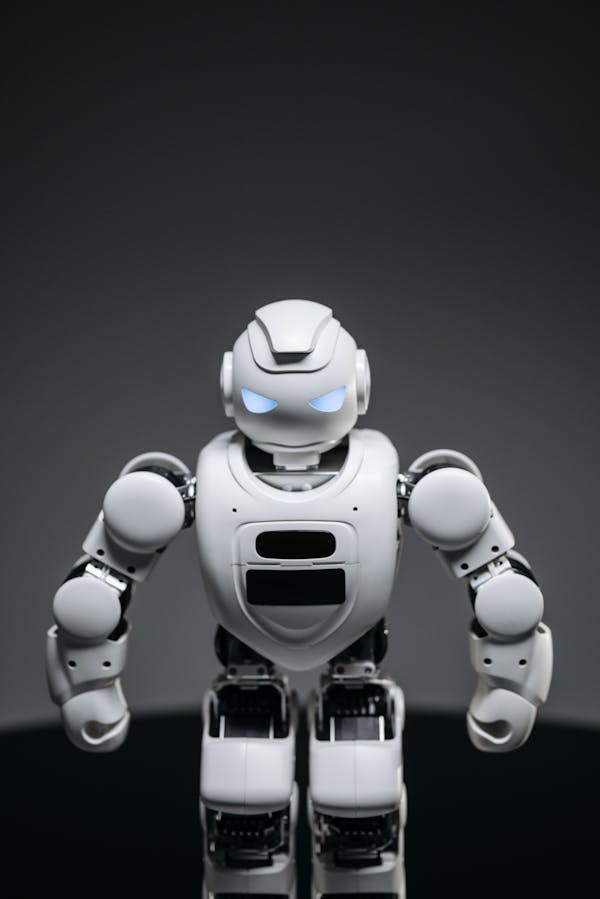
By the 1970s, a report from James Lighthill highlighted researchers’ underwhelming progress and led to a reduction in government funding. However, a brief boom in the 1980s reignited interest and investment. This was fueled by the success of expert systems and the use of a computer programming language called Lisp. These developments would eventually lead to modern AI technologies like deep learning and neural networks.
Generative AI
A generative AI system can create text, images or videos based on a prompt. Unlike machine learning algorithms, which require labeled data to recognize patterns, generative models can produce new content with no underlying structure.
Advances such as deep neural networks and breakthrough language models have driven the growth of generative AI. These technologies enable a range of use cases from chatbots to image creation to music and video generation.
For example, generative AI has helped business leaders write readable text and photorealistic stylized graphics for marketing materials. It can also help software developers create readable, functional code with ease. In biopharma, generative AI can generate new drug molecules to accelerate R&D cycles.
Reactive AI
Reactive AI operates solely on the basis of its immediate environment & does not rely on stored data. These machines can’t learn or imagine the past or future; they simply react to specific scenarios.
For example, a spam filter or Netflix recommendation engine are examples of reactive AI. IBM’s chess software Deep Blue is also a good example of a reactive machine.
Purely reactive AI systems are useful for certain tasks requiring fast, precise reactions to specific situations without the need for learning or adaptation. However, they are vulnerable to attack & can be fooled by hackers using fake data. Thankfully, new technology is constantly improving & refining purely reactive AI.
Limited memory AI
The most widely used type of AI today, limited memory AI incorporates the ability to store and use historical data in addition to preprogrammed information. This allows limited memory AI to make better decisions than reactive AI and improve over time.
For example, e-commerce and streaming services utilize limited memory AI to create personalized recommendations. Additionally, self-driving cars use limited memory AI to observe and analyze road conditions and experiences in order to better navigate.
Unlike reactive AI, limited memory AI does not retain past experiences or data permanently, but rather stores it for a short amount of time to inform future decisions. This is the type of AI that powers virtual assistants like Siri or Alexa and chatbots.
Safety and ethics
AI’s rapid rise has created opportunities for entrepreneurs, businesses, and regular users worldwide. It also presents a number of ethical concerns, including bias, discrimination, and threats to human rights.
Identifying and mitigating risks requires collaboration between consumers, technologists, developers, mission personnel, information management and classification, and civil liberties and privacy professionals. This includes determining the goal of an AI system and related risks and benefits.
Developers should use accurate, fair, and representative data sets to avoid bias in their AI. They should also share a plan for how to manage bias and hold themselves accountable for the AI’s performance. Confidential and sensitive personal, customer, or company information should not be shared with AI tools; this could violate privacy legislation or confidentiality agreements.
Legal issues
The legal landscape surrounding AI is constantly evolving and needs to reflect the pace of technological advancement. This area of law requires a high level of agility, as well as foresight into the potential consequences on people’s lives and their human rights.
For example, generative AI raises questions about copyright laws, such as who owns AI-generated content. Comedian Sarah Silverman, for instance, is facing multiple lawsuits from companies like Meta and OpenAI, accusing them of copyright infringement for using her protected works to train their AI tools.
Also, if AI is responsible for harming someone, it can be difficult to attribute liability when its decision-making process is opaque. This has raised demands for transparency and explainability (Bodo et al 2018; Lepri 2018).