AI is a broad term that can mean different things to different people. It’s a concept that can spark passionate, often heated debates.
At one end of the spectrum are those, like podcast hosts Hanna and Bender, who prick the balloons of over-inflated hype. At the other are those who believe we’re on the verge of unlocking something called superintelligence—a sci-fi technology that can do everything humans can. AI Is More Fun Now, But Not For Everyone.
Machine Learning
Streaming services use AI algorithms to improve search results and content recommendations for subscribers, while online retailers use them to tailor product offerings based on customer behavior. These technologies make it easier to find what you want and need quickly, improving efficiency and productivity.
These same AI algorithms help companies glean insights from mounds of data to increase operational efficiencies and gain competitive advantages. For example, in healthcare, AI is used for accelerated drug development, patient monitoring and information extraction from clinical notes. The technology is also used to detect anomalies among massive amounts of data and reduce human error in a variety of industries, including financial forecasting, optimizing energy solutions and user personalization.
As a result, more organizations are using AI to augment human performance rather than replacing them entirely. But this shift can bring challenges, as some people fear their skills will become obsolete or be misappropriated. This is why we need to ensure that artificial intelligence remains “human centered,” “inclusive” and accountable.
Natural Language Processing
Natural language processing (NLP) is an area of artificial intelligence that enables computers to understand and process human language using statistical, machine learning and deep learning models. NLP is used to perform tasks such as sentiment analysis, text classification, machine translation and chatbot development.
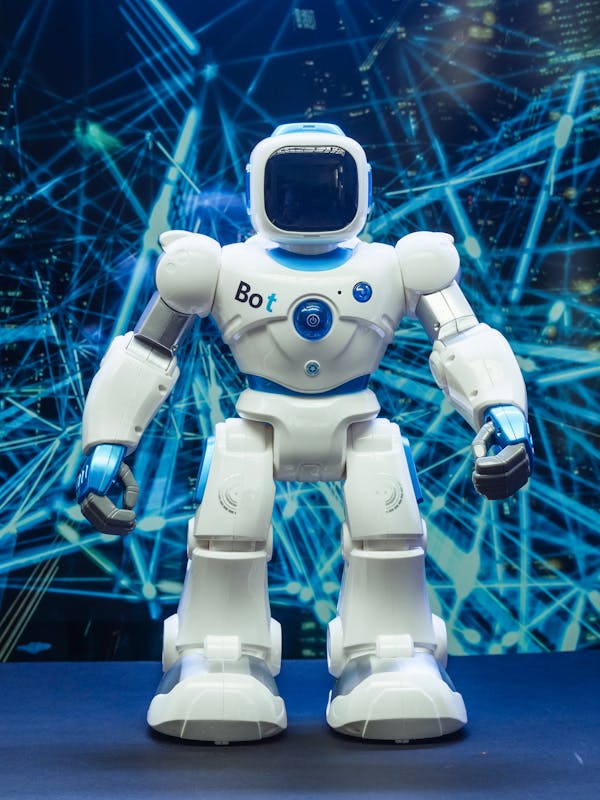
NLP algorithms use a combination of methods to identify and recognize phrases, words and sentence boundaries. Rule-based systems use pre-defined rules that look for punctuation and other markers to determine where one sentence ends and another begins, while statistical and neural networks models can automatically identify patterns in annotated data sets.
While NLP is still a work in progress, the technology has been improving rapidly, thanks to advances in recurrent neural network architectures that can crunch large data sets on accelerated hardware like GPUs. As more of us interact with computer systems using voice commands and typed text, NLP will become increasingly important in helping machines interpret and respond in the same way that humans do.
Generative AI
Generational AI, also known as deep learning, uses neural networks to create content and ideas autonomously in response to inputs or prompts. It’s fueled by data and has driven breakthroughs in computer vision, natural language processing and anomaly detection.
For example, GANs and variational autoencoders enable Google autocomplete to suggest possible search terms based on previous queries. These foundation models also power generative AI tools such as recurrent neural nets and deepfakes, which can be used to create fake images, video or audio to damage reputations, spread misinformation or make fraudulent financial transactions.
To prevent these dangers, organizations must implement responsible AI practices that include clear communication about how the model works, mark all gen AI outputs as “AI” and use an underlying architecture that supports a human-first design philosophy and includes a robust technical infrastructure with encryption protocols for data at rest and in transit, secure access control and regular security protocol assessments. It’s also important to incorporate local tasks that are too complex or time-consuming for generative AI.
Decision Making
Decision-making AI is software that learns and incorporates real-world experience into its algorithms. Unlike passive machines that perform mechanical or predetermined tasks, AI-enabled devices can analyze data instantly and make decisions with intention.
Using predictive analytics, AI can help companies avoid costly mistakes and identify opportunities for improvement. For example, in health care, AI can improve the effectiveness of treatments for individual patients by predicting which ones are most likely to succeed or fail.
It can also help financial professionals process high volumes of transactions faster and more accurately. This type of AI can improve human performance by eliminating manual processes, allowing employees to focus on higher-value activities. It can even replace some functions entirely. However, research by Workday found that 94% of business leaders believe it’s critical to use AI in partnership with humans for major strategy and decisions. Known as the human-in-the-loop approach, it allows companies to benefit from the speed and efficiency of AI while retaining control of important decisions.